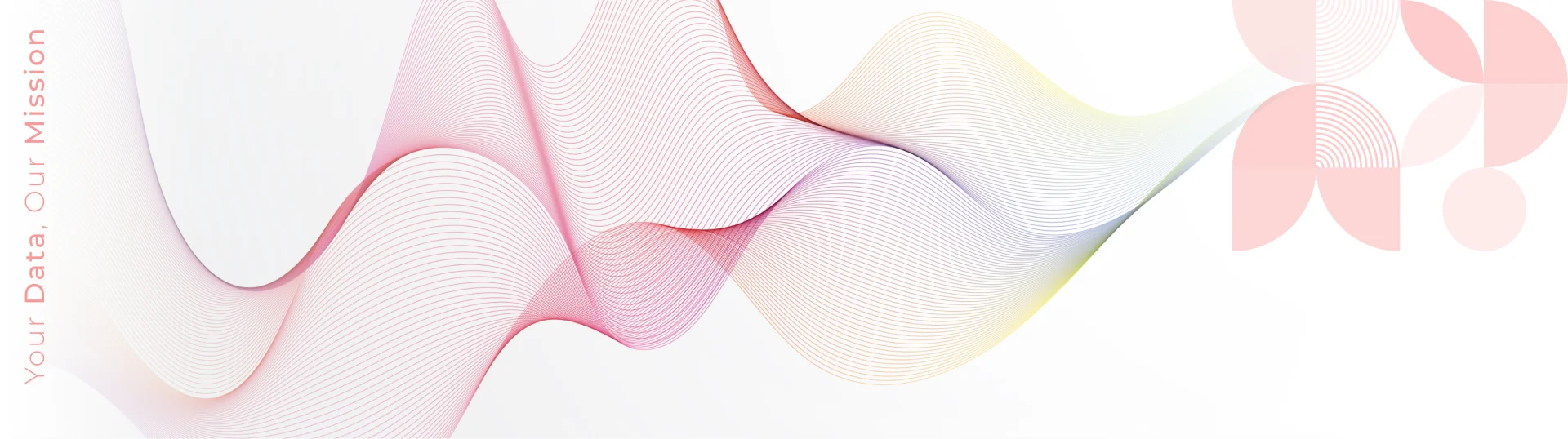
Want to Make Your AI Initiatives Successful? Focus on Data Diversity
Data is the new soil – when cultivated the right way, organisations can achieve results like never before. From understanding business process inefficiencies to better understanding customer behaviour, predicting maintenance schedules to optimising inventory, unearthing employee concerns to keeping pace with market changes – analysing the growing volumes of data has brought organisations to the forefront of success.
AI has a significant role to play in this. By connecting people, technology, and insights, AI is enhancing business analysts’ skillsets, enabling them to build unmatched revenue and customer lifetime value models.
However, many AI initiatives lack the finesse of a truly data-centric model. While many organisations blame it limited knowledge of AI technology, what ‘s really lacking is a focus on data diversity.
How AI models function
In a world where businesses are drowning under the sea of growing data, AI makes it possible for machines to take in data, perform human-like cognitive tasks, and recognise patterns while learning from experience. From playing chess to self-driving cars, virtual shopping experiences to fraud detection, identifying patterns in genes to automating processes – the scope of AI has expanded to include almost every aspect of the business.
By combining large amounts of data with fast, iterative processing and intelligent algorithms, AI interprets text and images, discovers patterns in complex data, and acts on those learnings. Using machine learning, neural networks, deep learning, natural language processing, cognitive computing, and computer vision, it sets the pace for fathering insights and automating tasks at an otherwise unimaginable rate and scale.
Why data diversity is important
Despite the profound ways in which AI can unearth insight from complex data, many organisations fail to achieve expected outcomes from their AI investments. In most cases, this is a result of data diversity issues. Since AI algorithms learn from experience, it is important for organisations to feed extremely diverse data sets to allow the models to output results that are all-encompassing and free of bias.
According to an article by Forbes, data diversity issues have caused AI algorithms by top IT giants to make several mistakes, including downgrading female job candidates, adopting racist verbiage, and mislabeling Congress members as criminals. Such mistakes bring about several legal repercussions. Also, failure to address them in time is bound to downgrade the accuracy of AI algorithms – making them deliver inaccurate and sub-par results.
Tips to ensure data diversity
Given how dependent modern organisations have become on AI to continuously analyse data to spot outliers and detect trends, they have a moral obligation to actively address data bias. Since AI models are not built with biases but arise due to the data they are fed with, the only way to address this issue is by diversifying data as much as possible to minimise bias propagation and amplification. Here are some tips to ensure data diversity:
- Build a team of diverse individuals: Have a team of people with diversified experience, backgrounds, ethnicity, race, age, and viewpoints to carry out data collection and preparation. This will not only ensure diversity in an academic discipline and risk tolerance but also in political perspective and collaboration style. A team with intellectual diversity can enhance creativity and productivity growth and improve the likelihood of detecting and correcting bias.
- Check the quality of data: Another way to alleviate bias inside data sets is to constantly check the quality of data that is being fed. Instead of feeding AI models with all the data that gets generated within an organisation, analysts need to check if the data is up to standard. If it is not, they can suggest a diversity of viewpoints that were missing.
- Constantly monitor results: Another important aspect of sustaining the effectiveness of AI initiatives is by constant monitoring of results. Although you can allow AI models to do most of the heavy-lifting analysis work, data scientists need to constantly monitor results and check for unusual distributions or highly correlated variables.
- Balance bias if required: No matter how hard organisations try to minimise bias, the truth is that it can never be completely eliminated. However, they can minimise bias by balancing it with proper attention and effort. By adjusting data sets or employing mitigation strategies, they can bring down the chances of bias and improve the accuracy of results.
When AI was first introduced, organisations were worried if the concept would work. Fast-forward to today, where AI has proven its capabilities in several different areas and sectors. The issue lies with avoiding bias in AI results. Since AI algorithms are built to automatically scan through millions of data sets to unearth insights, without any human intervention, feeding diverse data is the only way to enhance the quality of results. In addition, constant efforts towards prevention, removal, and mitigation of bias are essential to ensure AI continues to amaze the world with its unmatched capabilities and on-point insights.